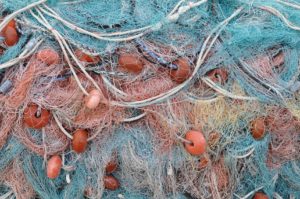
More than ever, the ability to use data for decision-making is critical to company success. Despite this knowledge, companies are still not fully empowering their employees with easy access to the data they need. According to Zhamak Dehghani, the founder of Data Mesh, we must start thinking outside of the box because the traditional approach to managing and collecting data is not sufficient any longer.
For decades, there has been a divide between operational and analytical data with ETL as the intermediary process to get data from operational systems into the analytical data warehouse. ETL, which has always been primarily in the hands of IT developers, is perceived as a bottleneck to delivering timely analytical data. Furthermore, dimensional data models are not well suited for machine learning models that have become essential.
To overcome this, the data lake emerged around 2010. The idea of the data lake is to store vast amounts of semi-structured data in object stores to allow various consumers to use the data according to their needs. But there are challenges with accessing heaps of data that have been dumped into the data lake without giving much thought to its organization and consequently the data lake did not live up to its potential.
With the proliferation of cloud providers, such as Snowflake, we have an immense number of tools at our disposal that should allow users to access their own data as they see fit. What is still missing is a paradigm shift in architecture, organization, and technology. The data mesh architecture has emerged as a new framework to help solve these missing pieces. It encompasses four principles that are elaborated in the sections below.
The Snowflake Data Cloud connects organizations and data teams with the data they need, when they need it, without silos or complexity. Snowflake has been built for ease of use, performance at scale, and governed data sharing, all features that are well aligned with the data mesh principles.
…
Read more here.